User:Rvuchkov/sandbox
In algebraic statistics the concept of maximum likelihood degree (ML degree) arises naturally as the number of complex solutions of the likelihood equations. The ML degree is bounded by the degree of the likelihood ideal.
Introduction[edit]
A parametric probability model for a discrete random variable is given by a map where is an open set and is the probability simplex . The model is , where and . The problem of maximum-likelihood estimation for a fixed data vector is to find a parameter that best explains the data vector, leading us to the problem of maximizing
- subject to
Equivalently maximizing the log-likelihood function
In the above definition if are polynomials in , the Zariski closure of is called an algebraic statistical model. To employ tools from algebraic geometry we let the domain of be extended to the complex numbers.
- such that
The Maximum Likelihood Degree[edit]
The maximum likelihood degree (ML degree) of a discrete statistical model is the number of complex critical points of the log-likelihood function.
- for generic data .
Equivalently the ML degree is the number of complex solutions to the system of equations
Where and is the sample size .
Example[edit]
The Hardy-Weinberg curve has ML degree 1.
Where is the parameter of a biased coin to land on tails. With these equations, we suppose that the coin is tossed twice; Then the equations represent the probability of a heads appearing. We can repeat the experiment times. Construct a data vector where is the number of times heads appear. Inspired by the MLE problem of estimating the unknown parameter by maximizing the following:
One may find it more convenient to work with the logarithm of the likelihood function. Furthermore there are many ways to maximize the log-likelihood function such as Lagrange multipliers and other optimization tools. For this particular example we do not need any such machinery.
After applying the Lagrange multipliers method for optimization the following polynomial (likelihood equation) is produced.
This is a polynomial of degree 1, which implies one complex solution. Thus ML degree is 1.[2]
This example is very interesting because a generic quadric has ML degree of 6. This implies that our example that was given here is of particular interest. In general solving the likelihood equations might be troublesome to do by hand and computer software such as Macaulay2, Singular, and Polymake, may be helpful.
Birch's Theorem for Toric Ideal[edit]
Let and be a vector of positive counts. The maximum likelihood estimate of the frequencies in the log-linear model is the unique-nonegative solution to the simultaneous system of equations:
and
Note that the number of complex solutions to is the ML degree. [3]
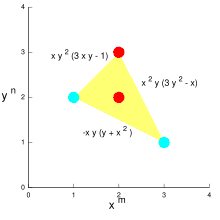
History[edit]
The ML degree is a more recent work which started with two papers: The maximum likelihood degree paper by [Catanese, Hosten, Khetan, Sturmfels] and Solving likelihood equations paper by [Hosten, Khetan, Sturmfels]. Both papers describe on the connection between ML degree and polytopes and Newton polytopes. [4]
References[edit]
- ^ Catanese, F., Hoşten, S., Khetan, A., & Sturmfels, B.. (2006). The Maximum Likelihood Degree. American Journal of Mathematics, 128(3), 671–697. Retrieved from http://www.jstor.org/stable/40067993
- ^ Huh, June; Sturmfels, Bernd. "Likelihood Geometry". aeXiv.
- ^ Drton, M., Sturmfels, B., & Sullivant, S. (2008). Lectures on algebraic statistics (Vol. 39). Springer Science & Business Media.
- ^ Hosten, S., Khetan, A., & Sturmfels, B. (2005). Solving the likelihood equations. Foundations of Computational Mathematics, 5(4), 389-407.
External links[edit]
- Home page of Twenty-First Holiday Symposium: Gröbner Bases and Convex Polytopes
- Home page of D. A. Cox, with several lectures on toric varieties